A deep dive into the ECB’s climate risk pricing research
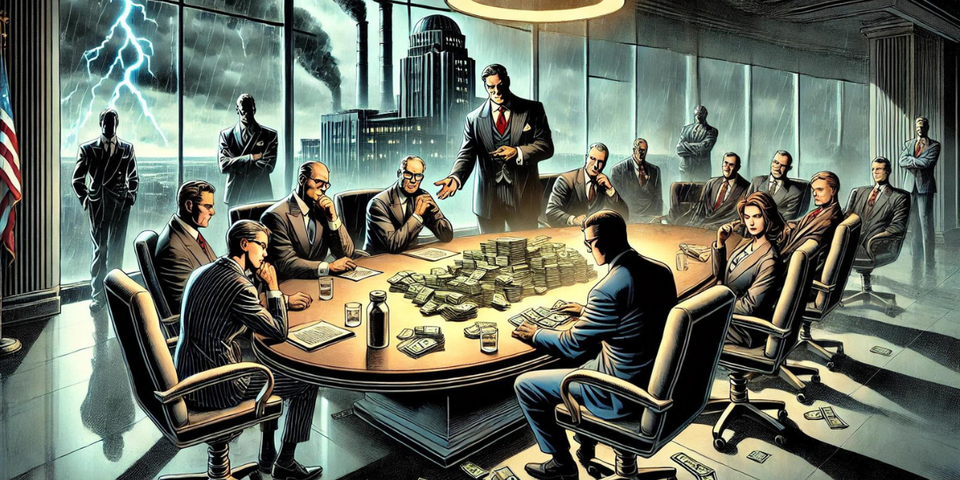
Much of the climate-related discussion generated by central banks is based on scenario analysis, a technique that invariably fails to tell us how financial risk actually manifests. Therefore, it’s a refreshing change when they release properly constructed empirical research on the topic, as the European Central Bank has. Nerds like Louie and I are compelled to pore over the results to try to work out whether and how our prior views should be updated.
The paper was written by Carlo Altavilla, Miguel Boucinha, Marco Pagano and Andrea Polo. I’ll refer to it as Altavilla et al (2024).
I thought Louie’s piece on Friday did an excellent job of highlighting the key takeaways. I should say that we’ve both sworn a blood oath that Unpacking Climate Risk will not be a mutual admiration society; we will always try to highlight situations where we agree and where we disagree. In this case, though, I fully support his arguments. I think I can build on Louie’s discussion without having to re-hash any of the key points made in Friday’s piece.
Here I’ll do three things in reference to Altavilla et al (2024): One, I’ll point out an alternative interpretation of the results; two, I’ll add some technical discussion of the models used; and three, I’ll discuss the monetary policy implications.
Technical
Altavilla et al (2024) finds that ‘dirty’ firms without a plan to get greener pay higher interest rates, which is highly encouraging. As Louie pointed out, in an important sense the exact cause of the elevated risk premium is less important than the reality of its existence. We all want global warming to be fixed and we think finance can play an important role. Louie also noted that the rate bump is fairly modest – there’s only a 0.14% average differential between ‘dirty’ and ‘green’ firms.
You often find this with studies based on data from financial markets. Because you typically have a lot of companies in the sample that are observed with high frequency, the datasets tend to be much larger than those studied in Stats 101 – in this case, the researchers had over 300,000 matched observations. With this much data, it’s possible to identify very subtle behavior. You can find results that are highly statistically significant even though they only perturb the modeled results (in this case, the risk premium) a tiny amount. If you see research using financial data that fails to discuss the economic significance of the findings, you should ask what the researchers are trying to hide.