Is climate risk cool for cats? Cat models, that is!
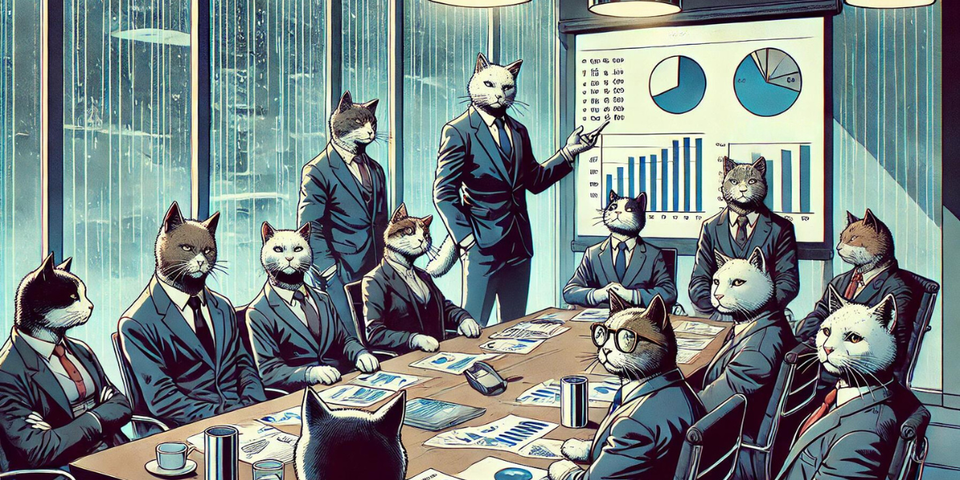
This is a free-to-view edition of Unpacking Climate Risk. Become a paying subscriber today to get articles sent direct to your inbox three times a week.
Many important decisions that are significantly impacting people’s lives are being made under the rubric of controlling climate risk. Folks are being denied mortgages and insurance, property valuations are rising and falling, and banks are being hauled over the coals for perceived shortcomings in their management of climate-related financial threats. In the near future, it is conceivable that banks will be required to raise additional capital to cover the perceived extra risk their supervisors say they face from climate change – restricting their ability to extend credit.
But an important question remains: is our response to the threat of climate change effective? Or are we just putting on an elaborate show, engaging in nothing more than performative greenwashing: “Can’t you see how seriously we’re taking this challenge?!? We’re building our entire business/economy around controlling climate-related risk!”
Most of the decisions referenced above are informed by the output from catastrophe ( or “cat” models). An insurer will use the insights from cat models to determine the likelihood that a property will suffer damage from a natural disaster and prompt a claim. This was what cat models were designed for.
However, more recently banks have started using cat models to classify new loans based on their physical risk profiles. Various companies, including insurers like Swiss Re, have packaged their cat models for use by banks and regulators and, to this end, have added forward estimates of disaster probabilities under a range of global warming scenarios.
It’s now possible to source, for example, flood or wildfire probabilities for specific locations up to a century in the future. If you need to know the likelihood of alluvial flood at 843 Hickory Lane, Smallville, Kansas in the year 2080 under the RCP 8.5 scenario, there’s about a million vendors who will happily help you populate your spreadsheet (for an appropriate fee, naturally).
But even the cat estimates for the present day produced by these models can vary dramatically from vendor to vendor. This issue was highlighted in a recent Bloomberg article. The authors looked at predictions from a range of models for Los Angeles County and compared the way they classified the risk of flood. They found dramatic disagreements – for a given location, one model suggests a high risk of catastrophe while others give the all-clear.
These disagreements must be frustrating for cat model users – which output should they believe? Bear in mind that we’re talking about current risk estimates here. We haven’t even got to the far murkier issue of 30- and 50-year scenario projections. I’ll leave this to a future article but I think you can guess what my stance will be.
Conflicting cat model outputs are even more frustrating for the end-users of financial services.
A homebuyer in Compton or Santa Monica may go to one bank and have their mortgage rejected on the basis of their physical risk exposure. But next door, the rival bank could welcome them with open arms.
It Never Rains in Southern California (In Houston It Pours, Man It Pours)
The Los Angeles example doesn't tell the full story. Bloomberg was able to compare different model estimates for the city, but without an “Actual Outcome” column you can’t really tell what’s going on.
Thankfully, Kousky, Palim and Pan (2020) have done this for us. They were able to source a somewhat unique dataset from Houston following Hurricane Harvey in 2017. Here’s how Kousky et al describe the key variable in their database:
“Our dataset contains home inspection information that can be used to measure flood damage. Fannie Mae’s contracted servicers conducted post-disaster home inspections on all delinquent loans. The inspections were conducted of the exterior of the property either from a car or a walk around the grounds.”
We can overlay the numbers from a cat model onto these inspection outputs and see how well the model is actually doing. The inspection used is a little cursory, perhaps, but at least we have actual, literal observations with which to validate the predictive performance of the model. I’ve reproduced (and slightly enhanced) Kousky et al’s Table 3 below, the format should be straightforward and very familiar to readers:
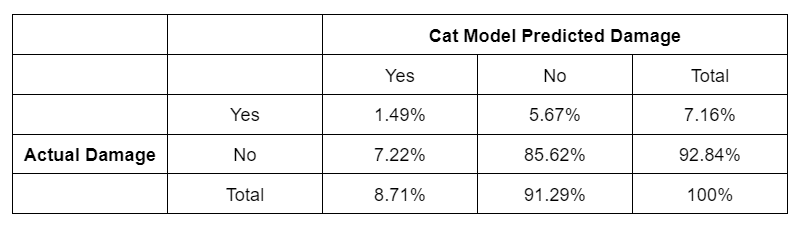
So the cat model predicted that 8.71% of properties would be damaged by a storm of Harvey’s magnitude, and 7.16% of properties were found to be damaged in reality. The model was a little pessimistic when viewed this way, but it didn’t do too badly.
But of the specific houses that were damaged by the storm, only 20.8% were correctly predicted by the model while 79.2% were erroneously given the all clear. Of the houses that were spared, 92.2% were correctly classified by the model. However, when the cat model predicted that damage would occur, 82.9% of the time it was found to be a false alarm.
If this was a cancer screening test, would the government mandate its use? Remember that bank regulators around the world are effectively requiring banks to use these models for credit risk assessment and stress testing.
Now, Kousky et al only looked at one cat model, it’s true. It’s possible that different models following different criteria could identify different risks faced by property holders in a given city. To clarify, suppose we have three cat models that don’t agree with each other and decide to declare the property “at-risk” if any of the models predict disaster. This might allow us to dramatically increase the prevalence of ‘true positives’.
But it would also, most likely, increase the rate of false positives just as dramatically. This would presumably make it much harder for anyone to qualify for a mortgage in the city!
What’s the solution to this dilemma? I’m not sure. It’s obviously bloody hard to predict which properties will be damaged when a storm rolls through. The old mantra of “more data, more research” applies just as it always has.
For insurers that are directly impacted by disasters, the models are obviously accurate enough for their specific purposes. Premiums are set high enough and claims are scrutinized appropriately for them to profit (most of the time) on their underwriting portfolios. If a particular region is too disaster-prone – and thus not profitable to insure – they can always exit the market and underwrite policies somewhere else.
For banks and others who are indirectly impacted by disasters, I’d argue the models are not accurate enough to boost risk-adjusted profitability. Kousky et al discuss this and conclude, for example, that cat models are not predictive of mortgage default. Despite this, if a bank wanted to use the models for their own private purposes, I would have no objection. I’d advise them not to bother – that it is a cost with no clear upside. But if they ignored me, no skin off my nose.
Much more troubling is how regulators are effectively moving to mandate the use of cat models by financial institutions. What is the state’s interest? They wouldn’t approve a Covid test with this level of effectiveness, so why is a climate risk test considered so necessary?
Perhaps it is just regulatory greenwashing. That’s my suspicion.
Member discussion