Why understanding Exposure At Default is critical to climate risk management
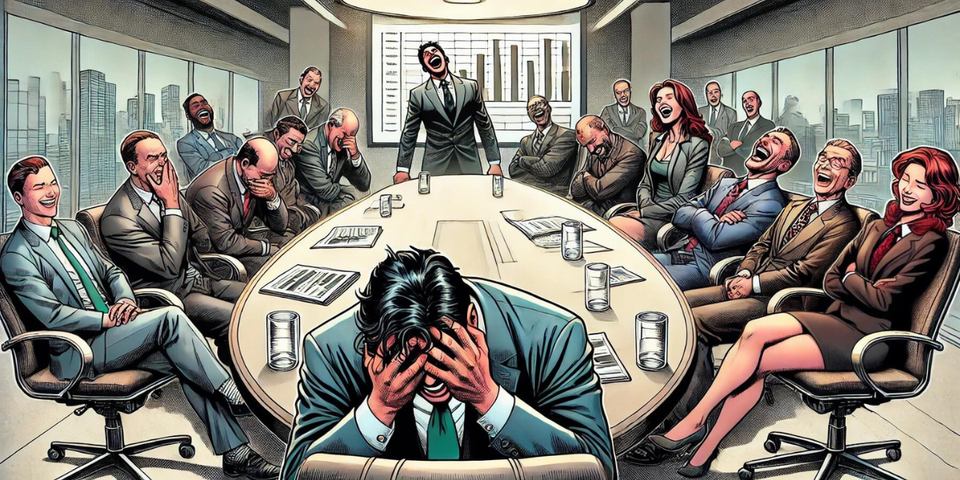
This article has been made free-to-view. Like what you read? Then consider buying us a coffee here👇
Expected credit loss is governed by a very simple formula:
EL = EAD * LGD * PD
EAD is exposure at default, LGD is loss given default and PD is the probability of default. LGD is normally expressed as a percentage, hence the multiplicative nature of the EL equation. It includes any and all anticipated administrative costs associated with winding down the exposure.
Think about a mortgage on the brink of foreclosure. Suppose the borrower has $5mn outstanding right now and that the current market value of the property is $4mn. The bank anticipates that it will lose a further $500,000 from delays in selling the asset, legal expenses, and other tasks required to recover the lost funds. In this case, EAD would be $5mn and LGD would be 30% (calculated as 1.5mn / 5mn).
I could write an entire article, perhaps a book, on how “default” is defined. Fundamentally, it occurs when a borrower stops paying back, but this can be indicated (or contraindicated) by many different circumstances. Most banks use a definition like 90-days past due as determinative of default, together with some administrative definitions like a borrower filing for bankruptcy. Even if this situation arises, the loan may still “cure” if the cause of the borrower’s financial distress proves to be temporary.
For our purposes here, it’s enough to recognize that borrowers may default some day, and that we can normally attach a probability to the occurrence of the event.
Risk analysts will devote most of their time to modeling PD, which is the most critical factor in triggering the recovery and loss recognition process. It also happens to be the component easiest to model, mainly because data on every loan, good or bad, provides the analyst with a signal about each loan’s creditworthiness at every stage of observation.
When we attempt to model LGD, by way of contrast, we must limit our attention only to loans that have previously defaulted. Such defaults, thankfully, are rare, but this means that even large banks often suffer too few defaults to allow LGD to be modeled with precision using statistical techniques. Using a broader industry-wide database eases this constraint, but understanding the behavior of loans that have previously defaulted remains very challenging. Many or most banks will use rough classifications based on loosely calibrated criteria to make assumptions about possible future LGDs.
Because the vast majority of risk assessments made by banks have a short time horizon – a year or three at most – EAD is normally thought of as a fixed constant for most bank exposures. The current outstanding balance determines the EAD, end of story.
One exception to this rule is for revolving products like credit cards. Unsecured borrowers are often very cunning and, if they get into difficulty, will try to max out their credit lines before the bank realizes what’s happening. For this reason, conservative analysts will generally assume that the credit line is fully utilized for EAD calculations. I’ve also worked with banks that have sought to identify and model the behavior of distressed borrowers, which gives them an additional point of control as the borrower stumbles toward default.
Even for revolving loans, it’s fair to say that current balance is a close approximation to EAD in virtually every case. So long as the focus is short term.
When climate-related financial risk started gaining traction as a concept, this way of thinking was adopted without question by financial regulators and, apparently, by many banks. Under many climate scenario analyses and stress tests, lenders must assume that their current portfolios will face a full slate of physical and transition risks. This is known as a static portfolio assumption.
Regulators use this purely for convenience. They wanted climate stress tests to ski in the tracks that had already been carved by annual capital adequacy exercises. In standard stress tests, the assumption is that the bad stuff happens right away at full throttle, so there’s no time for banks to actively manage their exposures.
However, with climate scenarios the situation is completely different – to the point where the traditional approach to expected loss calculation is laugh-out-loud silly.
Climate scenarios, even really severe ones, are assumed to creep up on us slowly. A typical study focused on physical risk will assume that temperature increases build up over 10, 20, 50 years or more, eventually leaving society in a perilous position. Studies of transition risk are of shorter duration – perhaps five or ten years – but the events are still assumed to be gradual and incremental in nature.
It makes no sense to imagine global temperatures moving from 1.5°C to 5°C tomorrow and it makes no sense to assume that all the necessary legislative changes needed to ensure net zero will be signed into law before we wake up in the morning. This makes the static portfolio assumption approach nonsense.
Under any scenario, banks will be able to anticipate, if imperfectly, what’s going on around them. They will have time to respond and find a way to either minimize losses or perhaps even find a way to profit from the events that are unfolding.
That’s kind of what banks do best.
A key consequence of this is that EAD cannot reasonably be assumed to be constant. We therefore need to understand the way climate scenarios may influence the exposures that banks might actually hold at the moment when climate-related defaults actually occur.
That means people are putting a huge amount of effort into calculating long-term expected losses for, say, that specific office building at 47 Main St Gotham City – an asset that is anticipated to experience a 10-fold increase in flood risk and a 20-fold increase in coastal inundation risk over the next 50 years.
This may be a fun and useful academic study if it’s done really well. In terms of practical relevance for the risks faced by a bank in 2024, there’s basically none. The building may be derelict or torn down or converted for an entirely different purpose by the time the physical peril manifests.
Analyzing the physical consequences of climate change for the specific geographical location of the Main St building is important, but not for the calculation of PD. Its main use should be to help the bank manage their overall exposure to the property as the climate situation worsens. Making strong assumptions about LGD and calculating EL only tells the bank how much they can expect to lose on something they ultimately won’t have any exposure to.
If the management of exposures is done really well, using data-driven and fully validatable methods, a bank may be able to reduce its exposure to the Main St building by 80, 90, perhaps even 100%. Sheesh, over a 30- or 40-year period, the owner of the increasingly flood-prone property will likely reduce their demand for debt finance just as fast or faster than the bank will reduce their supply.
In other words, the bank’s exposure may evaporate too quickly for their liking.
So if EAD → 0, EL also → 0 so long as PD * LGD doesn’t → 1 at a faster rate as temperatures or transition efforts increase without limit.
This limit theory does not tell us that EL will ever be zero under any particular scenario, but it does suggest that credit losses directly attributable to climate change are likely to be well contained as the situation worsens. It may be possible to contend that PD * LGD will rise faster than EAD falls, but this does not accord with my experience of the banking sector.
When climate stress testing became a thing, financial regulators tried to ride the coattails of “traditional” capital adequacy stress tests. This was a huge mistake because the exercises are like chalk and cheese: a sudden, surprise recession versus a slow worsening of a chronic crisis.
They should have done the hard yards and designed a new process from the ground up.
If they had, their prime focus would have been EAD in the context of dynamic portfolios. We all know that a doubling or tripling of PD or LGD is irrelevant if EAD is zero. After all, zero multiplied by a big number is still zero.
Working out the long term PD of a location that is material for a bank in 2024 is of virtually zero consequence. What would be much more useful is if banks were designing systems to control their EAD as climate change worsens.
Finally, notice that the empirical reality I have mentioned ad nauseum is fully consistent with the limit theory I discussed here. As climate-related risk builds, banks either manage their exposures carefully or don’t have to because demand for credit naturally wanes.
This is why I bet we don’t see banks experience heavy losses as climate change worsens.
Member discussion